Whether navigating self-check-out systems at the grocery store, passing through automated toll booths while driving, or interacting with virtual assistants that asks probing questions before directing us to customer care, the unescapable impact of AI automation has gradually altered our daily routines. This transformation brings about a dual effect on the economy and human workforce, yielding positive outcomes such as increased productivity alongside challenges like worker displacement.
Over the past sixty years, scientists and engineers have dedicated their expertise to advancing the capabilities of machines to perform pattern recognition and image/video analysis similar to human proficiency—a field commonly referred to as Computer Vision. Nevertheless, the recent emergence and widespread integration of technological innovations like ChatGPT, Gemini, and GPT-4 demonstrate considerable skill in tasks traditionally exclusive to human beings. Understandably, this heightened focus has ignited intensified discussions surrounding artificial intelligence’s impact on the workplace.
According to a study conducted by the MIT-IBM Watson AI Lab, the economic viability of automating vision tasks with “AI Exposure” in U.S. businesses is currently constrained by cost considerations. The research indicates that, at present, the majority of U.S. businesses would opt against automating such tasks, as the cost of developing, deploying, and maintaining a computer vision system outweighs the expenses associated with human labor. Specifically, the study found that only when automation costs are reduced significantly, or if AI is implemented through service platforms with a broader scale than individual organizations, would there be an increased inclination to adopt AI for vision-related functions. Noteworthy real-world instances supporting this concept include the development of a diamond classification tool by NavTech (Thompson 2021a) and a collaborative effort on a self-driving platform by NVIDIA (Thompson 2021b).
AI-Integration in the Workplace
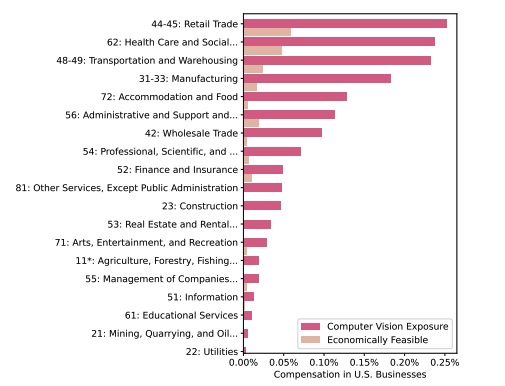
It’s important to note that the economic advantages of computer vision is predominantly concentrated within select sectors that exhibit the highest prevalence of vision tasks in the economy, thus yielding the greatest economic advantages. These sectors, namely Retail, Health Care and Social Services, and Transportation and Warehousing, stand out as key beneficiaries. Additionally, it is conceivable that these sectors experience notable returns to scale, evident in the presence of major industry players such as Walmart and Amazon, as highlighted by Svanberg et al. MIT’s research underscores the significant potential for AI to bring about job displacement, though the process is expected to unfold gradually. Consequently, this creates an opportunity for the implementation of effective policies and retraining initiatives to alleviate the potential impact of unemployment.
The cost of human labor versus computer vision automation is a complex and multifaceted consideration that depends on various factors, including the nature of the tasks, technological advancements, labor costs, and the specific industry.
Here are 7 key points to consider regarding automating tasks with computer vision:
- Repetitive and Predictable Tasks: Tasks that are repetitive, predictable, and involve a significant amount of data analysis or pattern recognition are often prime candidates for automation with computer vision. This includes tasks like quality control in manufacturing, visual inspection, and data extraction.
- Labor Costs: In regions where labor costs are high, companies may find it more cost-effective to invest in automation technologies. While initial setup costs for implementing computer vision systems can be significant, the long-term savings from reduced labor costs and increased efficiency may outweigh these initial expenses.
- Accuracy and Consistency: Computer vision systems can achieve high levels of accuracy and consistency in tasks that require precision and attention to detail. This can be especially valuable in industries such as healthcare, where accurate medical imaging analysis can lead to improved diagnostics.
- Complexity of Tasks: Some tasks involve a level of complexity that may be challenging for current computer vision technologies to handle effectively. However, advancements in artificial intelligence and machine learning continue to enhance the capabilities of computer vision systems, making them suitable for a broader range of tasks.
- Human Oversight and Collaboration: In certain scenarios, human oversight or collaboration with automated systems may be preferred. For example, tasks that involve creativity, critical thinking, or emotional intelligence are typically better suited for human workers. In these cases, automation with computer vision might be used as a supportive tool rather than a complete replacement.
- Regulatory Compliance: Some industries may have specific regulatory requirements or ethical considerations that impact the feasibility of automation. Companies need to ensure that any automated systems comply with industry standards and regulations.
- Maintenance and Adaptability: Automation systems, including those utilizing computer vision, require ongoing maintenance and adaptability to changes in the environment or task requirements. The cost-effectiveness of automation depends not only on the initial investment but also on the long-term maintenance and adaptability costs.
Ultimately, the decision to automate tasks with computer vision requires a meticulous cost-benefit analysis and consideration of the broader implications on the workforce and overall operations before implementation. For more workforce intelligence insights, subscribe at www.thedigitalhumanity.com or email [email protected].